- AstroFeather Tech Review
- Posts
- Conversational AI and Medical Chatbots Are Poised To Transform Healthcare
Conversational AI and Medical Chatbots Are Poised To Transform Healthcare
PLUS: Testing Generative AI For Automated Clinical Documentation

Image credits: Busakorn Pongparnit / Getty Images
Welcome to the 16th issue of the AstroFeather AI newsletter!
In the past week (or so), there have been several exciting stories about the use of generative AI (genAI), including automated ambient clinical documentation and conversational AI, to improve both the patient and clinician experience.
Elsewhere in the healthcare industry, hospital systems like Duke Health and UNC Health are working to bring genAI to the hospital setting, CareCloud is bringing genAI to the outpatient setting, and Amazon's virtual health clinic is available nationwide.
You'll find these and many more trending discussions in this week's issue. I hope you enjoy reading this week’s updates and if you have any helpful feedback, feel free to respond to this email, contact me directly on LinkedIn (@adideswilliams), or follow the AstroFeather LinkedIn page!
Thanks - Adides Williams, Founder @ AstroFeather
In this week’s recap (10 - 15 min read):
Update #1: Conversational AI and Medical Chatbots Are Poised To Transform Healthcare
Update #2: Testing Generative AI For Automated Clinical Documentation
Update #3: Trending Updates for AI in the Healthcare Industry
Update #4: Venture Capital Funding
Must-Read News Articles and Updates
Update #1. Conversational AI and Medical Chatbots Are Poised To Transform Healthcare.
The latest: With $59 million in new funding, a partnership with Cedars-Sinai Medical Center, and more than 3 million patients, K Health's medical conversational platform represents one of the early success stories in medical chatbot adoption, according to a Forbes report.

Image credits: K Health
At the core of K Health's medical chatbot is a language model that was initially trained on an anonymized healthcare dataset that included a wealth of medical information, such as 400 million medical records, 2 million hospitalizations, 500 million prescriptions, and over a billion lab results.
How it works: To access K Health's service, patients simply complete an AI assessment interview, where they provide their medical information and answer questions about their symptoms and medical history. K Health's chatbot generates possible conditions, and patients then have the option to connect with a provider for a virtual visit. Providers use their clinical judgment to make a final diagnosis, and comprehensive case management can include a variety of patient care interventions:
Access the service: Patients access the K Health service through a web or mobile app by entering their medical concern and providing demographic information.
AI medical interview: An AI medical "chat" conducts a dynamic interview, asking about medical risk factors, presenting symptoms, and collecting symptom-related information, and medical history.
Generate possible conditions: Based on the patient's information, the AI generates a list of possible conditions related to the patient's symptoms.
Virtual visit with a provider: Patients can opt for a virtual visit with a provider who reviews a detailed intake summary and the AI-generated differential diagnosis (DDx).
Provider’s clinical judgment: Providers use their clinical judgment to interview patients via text or video visit and make final diagnosis and treatment decisions based on their expertise.
Comprehensive case management: Finally, case management may include ordering tests, prescribing medications, recommending follow-up care, and making referrals to other providers, including face-to-face evaluation in primary or urgent care, specialty care, or the emergency department.
Results: K Health worked with academic researchers to conduct a retrospective study (not yet peer-reviewed) of more than 100,000 of its patient visits and found that (in general) clinicians agreed with K Health's AI-generated recommendations. Interestingly, the app seemed to be better at diagnosing some conditions than others:
Study results showed that human doctors and nurses agreed with one of the AI-recommended diagnoses ~84% of the time, and with the top-ranked AI diagnosis ~61% of the time.
The study also found high levels of accuracy for bladder infections, pink eye (conjunctivitis), and the common cold (upper respiratory infection), but was significantly less accurate for problems involving symptoms of skin rashes, asthma, and abdominal pain.
Yes, but: Not all users are satisfied with K Health's diagnostic platform. Negative customer reviews on the Better Business Bureau and Trustpilot tend to fall into the following groups, suggesting potential opportunities to improve user education about the chatbot's decision-making process and customer service:
Problems with scheduling an appointment.
Frustrated patients who couldn't get care online and were told to seek in-person care.
Patients who were denied antibiotics.
Driving the news: K Health is just one of several companies using conversational AI trained to answer medical questions and suggest possible diagnoses. Recent reports have highlighted that Google is testing its medical-focused language model, Med-PaLM 2, at the Mayo Clinic, and OpenAI’s ChatGPT is used in at least 6 hospitals and health systems for a variety of tasks. However, I wanted to highlight some conversational AI services that you may not have heard of, but that may be equally (or more) suited to the medical setting relative to ChatGPT/Med-PaLM 2:
Navina recently announced its generative AI assistant to help primary care providers navigate electronic health records (EHRs) more efficiently. Described as a "first of its kind," Navina's conversational AI platform is based on a proprietary "primary care language model" and uses natural language interactions to understand patients' health status and provide care recommendations based on patient data (such as lab results and discharge summaries) and clinical guidelines. The AI assistant can also perform administrative tasks, such as generating progress notes and referral documents, and assist with Hierarchical Condition Category (HCC) coding to estimate future healthcare costs for patients.
Hippocratic AI launched in May this year with $50 million in seed funding to develop a safety-focused large language model (LLM) designed specifically for healthcare. The technology is a text-generating model aimed at applications such as explaining benefits and billing, providing dietary advice and medication reminders, answering pre-operative questions, and onboarding patients. To assess its AI's "bedside manner," Hippocratic developed a benchmark to test the model for empathy, and it reportedly scored the highest across all categories of models tested, including GPT-4. The company has since announced a Founding Partner Program (including 10 health systems and digital health companies) and an additional $15 million in funding to further develop its healthcare specific LLM.
GenHealth AI, recently raised $13 million in seed funding to build its large medical model (LMM), which is specifically trained on medical data such as medical events and records, insurance claims, and medication costs, allowing it to outperform large language models (LLMs) in tasks such as evaluating patient care and financial claims. GenHealth AI plans to launch use cases for payers, pharmaceutical organizations, and providers, such as supporting risk adjustment, care management, and financial benchmarking.
Why it matters:
Healthcare-specific conversational AI services are part of a growing class of generative AI (genAI) technologies that hold great promise for shaping the future of healthcare, offering a wide range of benefits to patients, healthcare providers, and the healthcare industry.
For patients, medical conversational AI could improve customer service satisfaction by providing immediate answers to questions around the clock. Conversational AI services could also assist patients with medication management, providing timely reminders and valuable insights on dosage and side effects. For clinicians, medical chatbots could help save time by automating routine tasks, and generative search could provide better access to patient data, enabling quick information retrieval with summaries and insights.
On the other hand, we must also innovate and implement responsibly. It is important to recognize that genAI, while impressive, requires further testing to establish its safe use at scale in highly regulated domains such as healthcare. The adoption of genAI in healthcare must avoid pitfalls related to clinical bias, ensure the safety and reliability of AI models, and thoroughly test AI-generated recommendations before use in patient care scenarios.
Update #2. Testing Generative AI For Automated Clinical Documentation.
The latest: During the Amazon Web Services (AWS) Summit, the AWS team announced HealthScribe, a new generative AI (genAI) service that can be used to automatically capture and transcribe conversations between clinicians and patients.

Image credits: Amazon AWS
AWS HealthScribe uses a combination of speech recognition and genAI to transcribe and summarize conversations during patient visits, generate clinical documentation that can be uploaded to the electronic health record (EHR), save clinicians time on paperwork, and is (currently) designed to create clinical notes for general medicine and orthopedics.
How it works: It should be noted that AWS HealthScribe is not a standalone application, but a collection of AI services that can be integrated into a healthcare provider's existing clinical application to create their own AI voice scribes. The transcription process starts with the recorded audio of the patient visit, which AWS HealthScribe analyzes to create two files: a transcript file (with identified speakers and categorized dialogue) and a documentation insights file (with a summary of the discussion):
Transcribe conversations: During a patient visit, the patient-clinician conversation is recorded and then sent (via an API) to AWS HealthScribe, where it is transcribed (including timestamps for each dialogue) and analyzed.
Identify "who said what": During the analysis process, the transcribed conversations are analyzed to identify the individuals in the conversation, distinguishing between the physician and the patient.
Categorize transcribed dialogue: Transcribed conversations are organized into clinically relevant categories and summary sections such as "Assessment" and "Plan.
Extract key medical details: Conversation transcripts are further analyzed to identify clinically relevant information such as patient history, medication use, medical conditions, and any treatments discussed.
Clinical note summaries: Clinical note summaries are generated for key components of the clinical documentation process, including Chief Complaint (CC), History of Present Illness (HPI), Assessment, and Plan. Each sentence used in the AI-generated notes includes a reference to the original transcript.
Clinician review of clinical note: Finally, the summarized clinical note is sent back to the healthcare software vendor's clinical application for clinician review, editing, and finalization before being uploaded to the EHR.

Example application use of HealthScribe. Image credits: Amazon AWS
Results and early adoption: AWS has not publicly shared any research or technical reports that determine the accuracy rates of its HealthScribe service. The exact number of providers using HealthScribe is also not publicly available, but statements from early HealthScribe adopters, including 3M Health Information Systems, Babylon Health, and ScribeEMR, provide some indication of the (current) potential reach of ~300,000 providers:
3M Health Information Systems, which announced its collaboration with AWS to advance its conversational AI platform (including the 3M M*Modal automated clinical documentation system), which is currently used by "more than 300,000 clinicians."
Babylon Health, a population health company, said in a previous company announcement that its AI-powered platform supporting virtual care, Babylon 360, has a reach of "180 primary care providers and 1,000 specialty providers" through its existing partnership with FirstChoice Medical Group.
ScribeEMR, a virtual medical scribe provider, says its reach is "700+ satisfied providers" (at the time of writing), and Amazon has stated that ScribeEMR provides virtual medical office services to "hundreds of medical practices, hospitals, and health systems."
Yes, but: Accuracy remains a concern when using genAI-based tools for medical transcription and documentation. It is now well known that large language models (LLMs) such as GPT-4 tend to "hallucinate" and generate text that is incorrect, nonsensical, or fabricated. In fact, a Wallstreet Journal report highlights a similar generative scribing service, DeepScribe, as a cautionary tale of the potential accuracy issues that can arise, and AWS' HealthScribe FAQ notes the service's limitations:
DeepScribe, a GPT-4-based medical transcription service, was the subject of a recent Wallstreet Journal report (paywall) that interviewed current and former employees of the company, who noted that the AI often made mistakes, such as using incorrect medical terminology and listing medications that patients weren't taking. To correct these errors, the company reportedly relies on "200 human contractors to listen to medical conversations and revise records" to remove incorrect prescriptions and add correct billing codes.
The AWS HealthScribe FAQ notes that "the clinical notes generated by AWS HealthScribe are probabilistic and may not always be accurate due to various factors, including audio quality, background noise, [and] speaker clarity." The warning goes on to emphasize the importance of thoroughly reviewing HealthScribe-generated clinical notes for accuracy and sound medical judgment before using them in patient care scenarios.
Driving the news: As you might imagine, Amazon isn't the only vendor touting its AI note-taking services. Several companies, including Microsoft, Google, and Carbon Health, have recently introduced AI clinical documentation tools. In general, each of these services shares the following similarities: they all start with a recorded patient-clinician conversation and then, after transcription, use an LLM (or NLP)-based functionality to summarize the patient visit as a clinical note that is available for immediate clinician review:
Microsoft-owned Nuance launched its automated clinical documentation tool, Dragon Ambient eXperience Express (DAX Express), in March. Unlike AWS HealthScribe, DAX Express is a standalone application that integrates OpenAI's GPT-4 for its genAI capabilities and is currently only available in private preview for approximately 300 to 500 physicians, with general availability planned for early fall. It's also worth noting that Teladoc Health recently announced plans to integrate Nuance's DAX into its Teladoc Health Solo platform to automate the creation of clinical documentation during virtual exams.
Google Cloud partner Suki AI announced its Suki Assistant Gen 2 in April. Suki's AI assistant is a standalone app that uses a proprietary automatic speech recognition (ASR) model to transcribe conversations and a combination of proprietary and third-party LLMs to generate notes. While Suki AI hasn't publicly shared which LLMs it uses in its AI assistant, the partnership with Google leads me to believe that they may be using Med-PaLM 2 (Google's medical-based LLM that was recently made available to "select Google Cloud customers"). Suki Assistant Gen 2 is fully integrated with popular EHR systems, including Epic, Cerner, Athena, and Elation.
Carbon Health launched its “hands-free” clinical documentation tool in June. Carbon Health’s AI transcribing tool is a standalone app that transcribes audio using Amazon’s AWS Transcribe Medical, integrates GPT-4 to create summaries (and key takeaways) of the patient visit (like Nuance’s DAX Express), and is currently available to Carbon Health’s more than 600 clinicians.
Why it matters:
The prevalence of physician burnout has reached alarming levels, according to a 2022 advisory from the US Surgeon General. By 2034, the Association of American Medical Colleges (AAMC) predicts that the US could face a nationwide shortage of 37,800 and 124,000 physicians exacerbated by professional burnout related to the stress-inducing demands of physician EHR inbox work.
From the above examples, there are several hospital systems testing the use of automated ambient transcription services (specifically using genAI for clinical note generation) to reduce the administrative burden of EHR-related tasks. While it’s still early in the deployment cycle for some of these services, initial physician feedback (from pilot sites) suggests that automated transcription tools have a net positive effect on both the patient-clinician experience and medical documentation.
Update #3. Trending Updates for AI in the Healthcare Industry.
CareCloud and Google Cloud bring generative AI (genAI) to outpatient settings: CareCloud and Google Cloud have recently announced a landmark collaboration to bring genAI technology to the ambulatory healthcare setting. The partnership aims to improve the operational efficiency and capabilities of small and medium-sized healthcare providers. CareCloud will leverage Google Cloud's genAI services (including access to LLMs and Gen App Builder) to provide timely access to data and improve healthcare services for a broader range of clinics. The integration will enable physicians to ask complex questions, gain insights, and receive evidence-based recommendations based on patient data while complying with privacy laws.

Image credits: CareCloud
Amazon’s virtual health clinic goes nationwide: Amazon Clinic is now available to customers in all 50 states and Washington, D.C. This virtual healthcare marketplace offers video visits with board-certified physicians for more than 30 common health conditions (including pink eye and urinary tract infections). Users can access the platform through the Amazon Clinic website or mobile app, where they can connect with telemedicine partners, compare response times and prices across multiple telehealth provider groups, and receive treatment plans and prescriptions. The service is cash pay and available without appointments or insurance, depending on the customer's location.

Image credits: Amazon
Microsoft and Duke Health partner to deploy cloud technologies and genAI: Duke University Health System and Microsoft have announced a five-year partnership to explore generative AI and cloud technologies in healthcare. The collaboration aims to simplify and modernize Duke Health's IT operations using a secure Microsoft Azure cloud environment. The partnership will also focus on innovation and learning, leading to the development of AI-based tools for clinical care and the creation of a Duke Health AI Innovation Lab and Center of Excellence. Microsoft will help train Duke staff to foster a cloud-savvy IT workforce, and Duke will leverage Azure OpenAI services (which include ChatGPT and GPT-4 LLMs) to develop new tools that automate administrative tasks and personalize patient education resources.
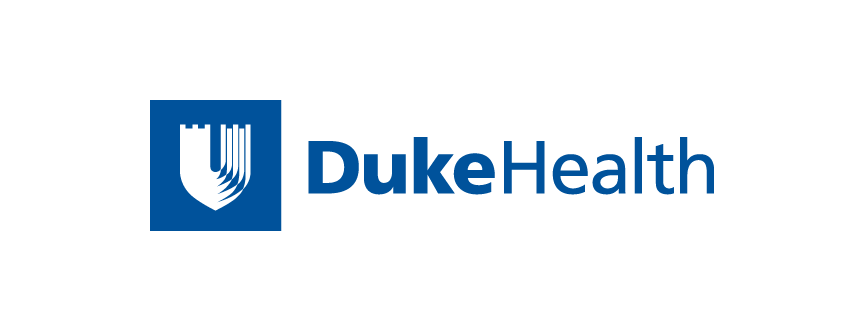
Image credits: Duke University Health System
UNC Health’s Epic InBasket pilot could inform future use of genAI in EHRs: UNC Health is now one month into its pilot of Epic's genAI tool, which drafts physician in-basket messages in response to patient inquiries. Early feedback from the pilot has highlighted the importance of continued physician engagement with the messages and the understanding that AI is intended to support, not replace, physician tasks. UNC Health is also working closely with Epic to incorporate user feedback and improve the overall design of the tool. As the pilot progresses, the health system will evaluate the impact on clinician efficiency and burnout. The success of the pilot could inform future AI innovations in EHRs by highlighting the importance of clinician engagement and how AI can streamline tasks.

Image credits: UNC Health
Ceribell received FDA clearance for its point-of-care electroencephalogram (EEG) headset system, along with AI/ML software to diagnose electrographic status epilepticus (ESE), a medical condition characterized by repetitive seizures that can lead to severe brain damage, are triggered without visible signs, and occur in patients with no history of epilepsy.

Image credits: Ceribell
Update #4. Venture Capital Funding.
BioNTech acquired InstaDeep for €500 million ($549 million) to strengthen its position in AI-driven drug discovery and the development of next-generation vaccines and immunotherapies for diseases with high unmet medical need.
Healthmap Solutions raised $100 million to support the expansion of its kidney health management program focused on the early detection of kidney disease.
RapidAI raised $75 million in a Series C round to support additional disease states with its AI-based imaging and decision support services. RapidAI currently offers AI and technology workflows for neurovascular, cardiac, and vascular disease.
GenHealth AI raised $13 million to further develop its large medical model (LMM), which is specifically trained on medical data (including medical events and records, insurance claims, and medication costs).
iotaMotion raised $12 million in a Series A round to accelerate the commercial expansion of its iotaSOFT Insertion System for robot-assisted cochlear implantation.
Thanks for reading this issue of the AstroFeather newsletter!
I’m always looking for ways to improve and would love to hear your constructive feedback about the format and content of the newsletter. You can reply to this email, and I’ll be sure to respond.
See you in the next issue!
If you enjoy AstroFeather weekly content, be sure to share this newsletter!
Adides Williams, Founder @ AstroFeather (astrofeather.com)
Reply